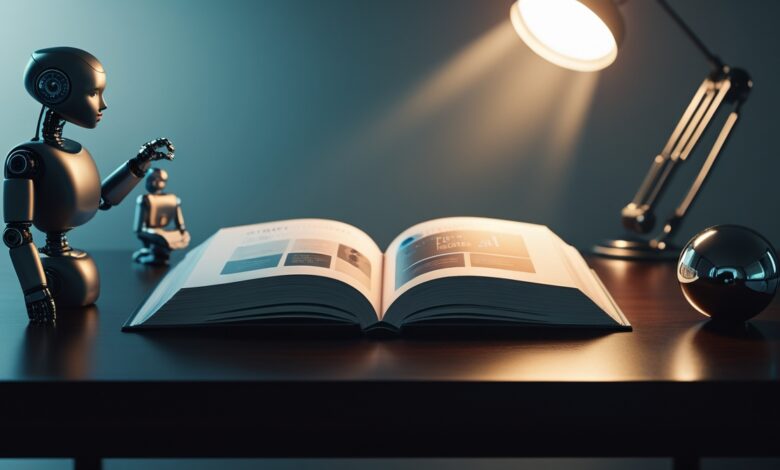
The Ultimate AI Glossary: Key Terms You Must Know
Artificial Intelligence applications are revolutionizing industries while changing our work methods and affecting everyday life. Artificial intelligence extends its reach from chatbots to self-driving vehicles throughout our world. The swift progress of technology brings with it an overwhelming amount of technical jargon. Business leaders and tech enthusiasts along with AI novices must understand essential AI terminology. This comprehensive AI glossary explains the essential concepts you need to understand.
1. Artificial Intelligence (AI)
Artificial Intelligence (AI) stands for machines that are designed to simulate human intelligence by thinking, learning and making decisions. The field of artificial intelligence covers multiple technologies such as machine learning, natural language processing and computer vision.
2. Machine Learning (ML)
Machine learning represents a branch of artificial intelligence where algorithms learn from data patterns to make predictions or decisions without explicit programming. Machine learning provides the foundation for recommendation systems and fraud detection alongside other applications.
3. Deep Learning
Deep learning represents an advanced segment of machine learning which employs multi-layered neural networks to process complex data sets. Deep learning has enabled major breakthroughs in both image and speech recognition.
4. Neural Networks
Neural networks function as pattern recognition algorithms based on human brain inspiration. Each layer in neural networks contains nodes or neurons that handle data processing before sending it to subsequent layers.
5. Natural Language Processing (NLP)
Natural Language Processing provides machines with the ability to comprehend human language and produce language-based responses. The technology powering Siri and Alexa virtual assistants extends to chatbots and translation applications.
6. Computer Vision
The field of computer vision develops systems that allow machines to understand and analyze visual data from the world including images and videos. The main applications for this field are facial recognition systems, medical image processing, and self-driving cars.
7. Algorithm
AI systems use algorithms which consist of specific rules and instructions that enable them to learn from data and solve various problems. An algorithm functions as the decision-making blueprint for AI systems.
8. Big Data
Big data describes massive datasets which researchers examine to discover trends and gain valuable insights. Large datasets function as fuel for AI systems to enhance their accuracy and performance.
9. Supervised Learning
In this machine learning approach algorithms receive labeled data which allows them to learn correct output pairings for given inputs. The objective is to develop a method that associates inputs with their corresponding outputs.
10. Unsupervised Learning
Unsupervised learning provides algorithms with raw data and does not specify the actions to perform on it. The system autonomously detects patterns and structures by organizing data points that share similarities.
11. Reinforcement Learning
In machine learning reinforcement learning agents learn which actions to take through trial and error by receiving rewards or penalties for their actions. It’s commonly used in robotics and gaming.
12. Chatbot
A chatbot represents an artificial intelligence program that enables human conversation simulation. Chatbots find extensive application across customer service functions as well as marketing activities and e-commerce platforms.
13. Generative AI
Generative AI describes algorithms which are capable of producing original content including text documents, visual imagery, musical compositions and programmatic code. Examples include OpenAI’s ChatGPT and DALL-E.
14. Bias in AI
AI systems demonstrate bias when their output becomes unbalanced because of incorrect assumptions or insufficient data. AI applications need bias correction in order to maintain accurate and fair operations.
15. Explainable AI (XAI)
Explainable AI works to ensure that the decision-making processes of AI systems remain clear and interpretable to human users. Building trust and accountability in AI systems depends on this fundamental requirement.
16. Internet of Things (IoT)
IoT represents the network that connects physical devices to the internet so they can both gather and exchange information. Through the integration of AI with IoT systems smarter decision-making and automation capabilities become possible.
17. Edge AI
Edge AI allows devices such as smartphones or sensors to process AI algorithms locally instead of using cloud-based servers. This reduces latency and improves real-time processing.
18. Turing Test
The Turing Test was introduced by Alan Turing to assess whether a machine can demonstrate intelligent behavior that cannot be distinguished from human behavior.
19. Robotics
The discipline of robotics merges artificial intelligence with engineering principles to develop machines that can operate independently or with minimal human supervision. Examples include industrial robots and drones.
20. Autonomous Systems
Self-governing systems operate independently to execute tasks without needing human control. Self-driving cars and automated warehouses stand as key instances.
21. Cognitive Computing
Cognitive computing strives to replicate human thinking patterns when faced with complicated scenarios. Healthcare diagnostics and financial analysis benefit from cognitive computing applications.
22. Predictive Analytics
Predictive analytics applies artificial intelligence alongside statistical algorithms to project future results using past data records. Multiple industries including marketing and finance as well as supply chain management depend on this technology.
23. Sentiment Analysis
Sentiment analysis is an NLP technique that identifies the emotional tone present in written material. Businesses apply sentiment analysis to assess customer opinions and feedback.
24. Transfer Learning
Transfer learning utilizes knowledge from one task to improve performance on a different yet related task. It’s a time-saving technique in machine learning.
25. AGI (Artificial General Intelligence)
AGI defines the capability of machines to achieve human-level understanding and application of knowledge across multiple diverse tasks. It’s still a theoretical concept.
Why This Glossary Matters
The ability to understand these terms is essential to navigating today’s AI-driven environment. This glossary provides essential information for anyone who wants to adopt AI in their business or stay updated with the latest tech trends.
Final Thoughts
Artificial Intelligence has arrived and continues to develop at a rapid pace. Mastering these key terms will enable you to effectively journey through the AI landscape and unlock its potential. Add this glossary to your bookmarks and return to it while you explore the field of artificial intelligence more thoroughly.